Chapter 5 Accountability
5.1 The increasing AI Autonomy
As more and more AI-enable autonomous decision-making systems are further developed and used, including ones that support life-critical scenarios, it will be important to create policy outlining where responsibility falls if things do go wrong.
To start with, watch the following short video delivered by Professor Virginia Dignum from Delft University of Technology, discussing 3 aspects of autonomy in intelligent systems, the ethics and impacts of these systems - and finally about the prospect of self-improving AI and superintelligence.5.1.1 Case study: self-driving cars
A self-driving car, also known as an autonomous vehicle (AV), connected and autonomous vehicle (CAV), driverless car, robot car, or robotic car, is a vehicle that is capable of sensing its environment and moving safely with little or no human input. AVs represent a major possibility for artificial intelligence applications in transport.
Watch the following short video from Associate Professor Nico Larco (University of Oregon and Director of the Urbanism Next Research Initiative), sharing his insights on how autonomous driving systems will open our eyes to a whole new way of thinking and how the world we live in could be fundamentally transformed.
Self-driving cars are already cruising the streets today.
And while these cars will ultimately be safer and cleaner than their manual counterparts, they can’t completely avoid accidents.
What are the considerations and how should the car be programmed if it encounters an unavoidable accident?
Discussions on the ethics of autonomous vehicles tend to focus on issues like the “trolley problem” where the vehicle is given a choice of who to save in a life-or-death situation.
Swerve to the right and hit an elderly person, stay straight and hit a child, or swerve to the left and kill the passengers? These are important questions worth examining (moralMachine?).
Watch the following video on the ethical dilemma of self-driving cars:
Activity: The Moral Machine Platform
In this activity, we are going to explore a few moral dilemmas on the design of self-driving cars, through the Moral Machine Platform, developed by MIT. Please visit the Moral Machine Platform. Three main functional interfaces can be accessed from the website (see, the menu bar):
- Judge: you will be presented with randomly selected moral dilemmas that a machine is facing. You are outside of the scene and have control over choosing what the self-driving car should do. You may proceed from scenario to scenario by selecting the outcome you feel is most acceptable by you. Upon finishing all the scenarios, you will see a summary of the aggregated trend in your responses in the session of the game you played, compared to the aggregated responses of others.
- Design: with this functionality, you can create a new scenario yourself.
- Browse: This interface enables you to view the scenarios you and other users of this platform have created.
For more details, please visit “View Instructions”: http://moralmachine.mit.edu/
5.1.2 Case study: Boeing 737 Max crashed twice
Airplane makers like Boeing and other makers of planes and cockpit-automation systems for some time have believed more-sophisticated systems are necessary to serve as backstops for pilots, help them assimilate information and, in some cases, provide immediate responses to imminent hazards.
The Boeing 737 MAX, which was announced in 2011 and entered service in 2017, is a narrow-body aircraft series manufactured by Boeing Commercial Airplanes as the next generation of a tried-and-tested workhorse of consumer aviation. The model has new more fuel-efficient engines and updated avionics and cabins, would have longer range, have a lower operating cost, and have enough in common with previous models so that pilots could switch back and forth between the two with ease.
In March 2019, aviation authorities around the world grounded the Boeing 737 MAX passenger airliner after two new airplanes crashed within five months, in October 2018, and March 2019, killing all 346 people aboard. After the first accident, Lion Air Flight 610 on October 29, 2018, investigators suspected that a new automated flight control, the Maneuvering Characteristics Augmentation System (MCAS), repeatedly forced the aircraft to nosedive. Boeing had omitted MCAS information from flight manuals and pilot training. In November 2018, Boeing and the U.S. Federal Aviation Administration (FAA) sent airlines urgent messages to emphasize a flight recovery procedure, and Boeing started redesigning MCAS. In December 2018, studies by the FAA and Boeing concluded that MCAS posed an unacceptable safety risk. On March 10, Ethiopian Airlines Flight 302 crashed, despite the crew’s attempt to use the recovery procedure. The airline grounded its MAX fleet that day.
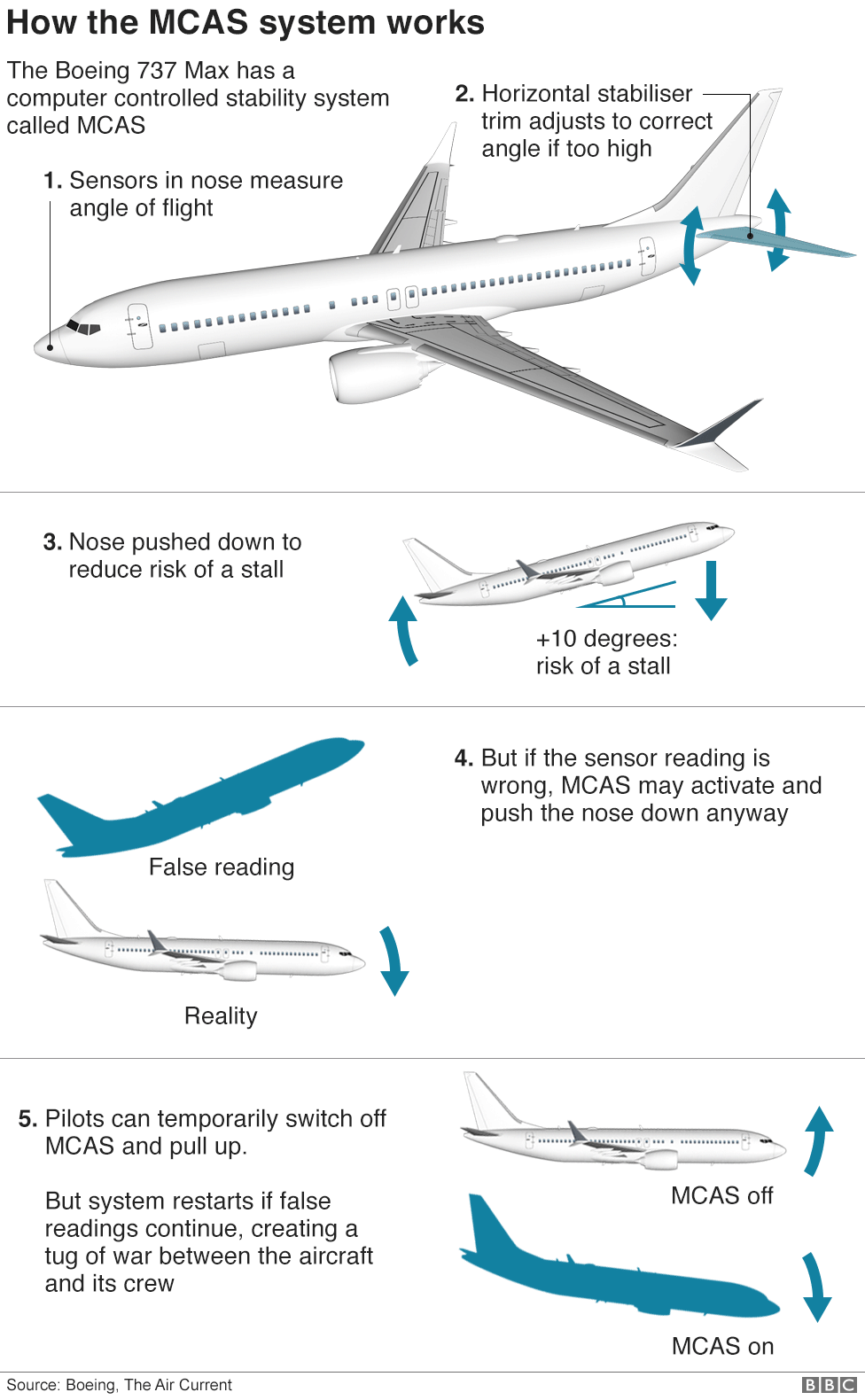
How the MCAS system works
DISCUSSION
- What lessons we’ve learned from the Boeing 737 MAX crashes?
- Who should be responsible?
5.2 Artificial Intelligence and Human Accountability
The developments in AI autonomy rapidly enabling these systems to decide and act without direct human control. Greater autonomy must come with greater responsibility, even when these notions are necessarily different when applied to machines than to people. As an AI system has no moral authority, it cannot be held accountable in a judicial sense for its decisions and judgments. As such, a human must be accountable for the consequences of decisions made by the AI. According to (main?), one of the core principles for AI is Accountability:
Accountability
People and organizations responsible for the creation and implementation of AI algorithms should be identifiable and accountable for the impacts of that algorithm.
In general, human accountability refers to the need to explain and justify one’s decisions and actions to its partners, users, and others with whom the system interacts (artOfAIDesign?). In the context of AI, accountability has a close relationship with transparency, since the ultimate goal of transparency measures are to achieve accountability. To ensure accountability, decisions must be derivable from and explained by, the AI algorithms used.
To foster human trust in machines and AI systems, we need to ensure that these AI systems are designed responsibly, such that we will be able to explain and justify decisions and understand the ways systems make decisions and to the data being used. Besides, there must be a clear chain of accountability for the decisions made by an automated system, i.e., answering the question of “Who is responsible for the decisions made by the system?” Responsibility refers to the role of people themselves and to the capability of AI systems to answer for one’s decision and identify errors or unexpected results. As the chain of responsibility grows means are needed to link the AI systems’ decisions to the fair use of data and to the actions of stakeholders involved in the system’s decision (artOfAIDesign?).
Watch the following talk from Joanna Bryson (Professor at Bath University), to learn more about Artificial Intelligence and Human Accountability:
5.3 Responsible AI
AI-enabled autonomous and intelligent systems are specifically designed to reduce the necessity for human intervention in our day-to-day lives. As the use and impact of these systems become pervasive, there is also an increasing need that a responsible approach is taken to the design and implementation to ensure the safe, beneficial and fair use of AI technologies, to consider the implications of moral decision making by machines, and the ethical and to define the legal status of AI (artOfAIDesign?).
These are further beyond simply reaching functional goals or addressing technical problems, it should ensure that these systems must be developed and should operate in a way that is beneficial to human being and the environment. Several initiatives are aiming at proposing guidelines and principles for the ethical and responsible development and use of AI (see e.g. IEEE Ethically Aligned Design report (ethicallyAlignedDesign?), the OECD Principles on AI, the Ethics guidelines for trustworthy AI (ec2019ethics?), just to cite a few).
Making AI systems responsible is much more than the ticking of some ethical `boxes’ or the development of some add-on explanatory features to facilitate human understanding of machine decisions. It requires the participation and commitment of all stakeholders, and the active inclusion of all of the relevant society. This means training, regulation, and awareness.
Watch the following guest lecture delivered by Professor Virginia Dignum from Delft University of Technology on responsible AI.
5.3.1 Case study: Uber fatality unveils AI accountability issues
The following case study is extracted from Chapter 4.4.1 (main?):Automated vehicles
In 2018, an Arizona pedestrian was killed by an automated vehicle owned by Uber. A preliminary report released by the National Transportation Safety Board (NTSB) in response to the incident states that there was a human presence in the automated vehicle, but the human was not in control of the vehicle when the collision occurred. There are various reasons why the collision could have occurred, including poor visibility of the pedestrian, lack of oversight by the human driver, and inadequate safety systems of the automated vehicle. The complexities of attributing liability in instances of collisions involving automated vehicles are well documented. In this case, although the legal matter was settled out of court and details have not been released, the issue of liability is complex as the vehicle was operated by Uber, under the supervision of a human driver and operated autonomously using components designed by various other tech companies. Following their full investigative process, the NTSB will release a final report of the incident identifying the factors that contributed to the collision. The attribution of responsibility in regards to AI poses a pressing dilemma. There is a need for consistent and universal guidelines, applicable across various industries utilizing technology that can make decisions significantly affecting human lives. In addition, policies may provide a universal framework that aids in defining appropriate situations where automated decisions and judgments may be used.